Let's face it: data is the new oil. It is a valuable commodity constantly mined in this digital age. As data grows in size and complexity by the day, it requires increasingly sophisticated efforts to sift through it all. That's where AI data analysis comes in. Deploying the power of artificial intelligence, AI-led data analysis turns vast amounts of data into valuable insights. Think of it this way - if data is indeed oil, then AI is the refinery.
Jump to a section:
💡 Understanding AI Data Analysis
🔃 Integrating AI into Data Analytics Processes
👍 6 Benefits of using AI for Data Analysis
🔮 AI’s Role in Future Data Analytics Trends
⚙️ Top 7 AI Tools for Data Analysis
Artificial intelligence has revolutionized data analytics, marking a significant paradigm shift in our approach to processing and interpreting large datasets. Gone are the days of manually analyzing spreadsheets and databases. AI has brought speed, accuracy, precision, and automation to the table, empowering organizations like never before to make informed, data-driven decisions. With this new era of AI-enabled innovation and strategic foresight, organizations equipped with AI-driven analytics are poised to lead.
ProServeIT, a certified Microsoft Solutions Partner in Data & AI, leads the way in leveraging AI data analysis tools to transform businesses in the digital age. Let's explore how AI deciphers the world’s data, byte by byte.
Through this blog, we explore what AI data analysis is, why it matters, the best AI tools for data analysis, the integration and impact of AI on data analysis workflows, and what exactly the future of AI data analysis looks like. Along the way, we even discuss how ProServeIT can help companies in their digital transformation journeys, with AI leading this change.
Understanding AI Data Analysis
Data analysis is the process of inspecting, cleaning, transforming, and modeling data to discover useful information, draw conclusions, and support decision-making. It involves various techniques, such as statistical methods, machine learning, and visualization, to extract meaningful insights from raw data. But what happens when you add artificial intelligence to the mix? How does it serve individuals and organizations in today's world? Let's find out.
What is AI Data Analysis?
In simple terms, AI data analysis is the process of using artificial intelligence to analyze and interpret data and extract useful insights from it. Leveraging machine learning algorithms, deep learning, natural language processing and other techniques, this technology streamlines the tasks of cleansing, examining, and modeling both structured and unstructured data.
AI in analytics goes beyond merely identifying ‘what happened.’ It delves deeper to uncover the ‘why’ behind events.
Imagine a retail company wanting to optimize its stock levels and reduce waste. By deploying AI data analysis, the company can analyze past sales data, customer behavior, and trends in real time. This application of artificial intelligence allows the business to predict future sales with remarkable accuracy, enabling them to adjust their inventory accordingly.
AI Data Analysis Techniques
AI data analysis uses various techniques to analyze and interpret large datasets. These include:
-
🖥️ Machine learning: a subset of AI that enables computers to learn from data without explicit programming, improving over time. In fact, machine learning is what powers personalized recommendations on streaming services.
🤖 Deep learning: a type of machine learning that involves training artificial neural networks on big datasets through unsupervised learning. Deep learning is used in facial recognition systems to enhance security and personalization features in technology.
🗣️ Natural language processing (NLP): a branch of AI that allows computers to interpret, understand, and generate human language. For instance, NLP enables virtual assistants to understand and respond to our voice commands.
📷 Computer vision: the use of algorithms to enable machines to recognize and interpret visual data.
🧠 Cognitive computing: a technology that simulates human thought processes using machine learning algorithms.
Why is AI Crucial in Today's Data Analytics?
The importance of AI in data analytics can be summarized in three words - speed, accuracy, and scalability. With the exponential growth of data collection, traditional methods of analysis fall short in terms of speed, efficiency and accuracy. On the other hand, AI-powered tools are faster and more accurate than human analysts, capable of processing and analyzing vast amounts of data in a fraction of the time. Moreover, AI can continuously learn and improve its capabilities with minimal human intervention, making it highly scalable for growing datasets. This pivotal role of AI underscores its importance and positions it as indispensable in the modern data analytics toolkit. Indeed, research by Gartner indicates that due to the influence of disruptive artificial intelligence (AI) technologies, 61% of organizations find themselves compelled to transform or reevaluate their data and analytics (D&A) operating models.
From predicting customer behavior in marketing to diagnosing diseases in healthcare, AI data analysis is transforming industries and shaping the future. A prime illustration of this transformation is evident in FedEx's operations. According to an article by VentureBeat, FedEx tracks data from its 18 million daily shipments across 220 countries. This vast repository of information fuels a range of analytics, predictive modeling, and initiatives based on AI and machine learning. The company meticulously analyzes every detail of a package's journey, including environmental conditions and weather, to design innovative customer-facing solutions that provide instant value.
6 Benefits of using AI for Data Analysis
The benefits of AI data analysis are multifold. Here are some ways in which organizations benefit by incorporating artificial intelligence into their data analysis process:
-
⚡ Faster insights and greater efficiency: AI-powered tools can analyze and interpret vast amounts of data at lightning-fast speeds, providing businesses with real-time insights. Furthermore, AI can enhance efficiency by swiftly retrieving commands and recommending alternative methods for data analysis. This is particularly beneficial when navigating through diverse data analysis libraries.
✅ Increased accuracy: Unlike humans, AI is not prone to human error and bias, allowing for more accurate data analysis. If analytics results deviate from expectations, AI can investigate the reasons behind it. It can even identify patterns, trends, and correlations that might otherwise go unnoticed.
🌐 Democratized Data: Through the power of AI and its application in natural language processing (NLP) within chatbots, access to data is becoming more democratized. This advancement enables professionals who are not experts in data to analyze vast datasets and derive important insights, without the necessity of mastering complex data science terminology.
💰 Cost savings: Automating data analysis allows organizations to conserve time and resources, previously expended on manual effort. AI streamlines the process by generating reports automatically, removing the necessity for manual compilation following each analysis.
📈 Enhanced scalability: As mentioned earlier, AI can continuously learn and improve its capabilities, making it highly scalable for growing datasets.
🎯 Better decision-making: With faster and more accurate insights, organizations can make data-driven decisions with confidence, leading to better outcomes.
As AI technology continues to advance and evolve, its impact on data analysis will only continue to grow. So, whether you are a small startup or an established corporation, incorporating AI into your data analysis process can help take your organization to new heights.
Integrating AI into Data Analytics Processes
AI has revolutionized the data analytics landscape by enabling real-time, predictive insights that were previously unattainable, substantially enhancing decision-making processes. Its integration facilitates a deeper understanding of complex data patterns, making data more accessible and actionable for businesses and organizations. Let's dive into its impact on workflows and its synergy with data analytics.
How AI Transforms Data Analysis
The integration of artificial intelligence into data analytics workflows has been unprecedented, especially regarding decision-making and business intelligence. Its proactive approach supports more informed strategic planning, risk management, and opportunity identification. The democratization of data analysis, facilitated by AI’s capability to present complex data insights in an understandable format, empowers a wider array of stakeholders to make evidence-based decisions. Here are a few ways in which AI impacts data analytics workflows:
-
🧹 Automating Data Cleansing and Preparation: AI-driven tools have revolutionized data cleansing and preparation by automating both routine and complex tasks, allowing for the rapid processing of large datasets with minimal human intervention. This automation, especially through Machine Learning (ML) algorithms, has significantly reduced the time and resources needed for traditional data analysis, which previously required extensive human effort to collect, clean, and prepare data before any analysis could commence.
🔮 Predictive Modeling: AI-powered algorithms can analyze large datasets, identify patterns, and make accurate predictions in a fraction of the time it would take traditional methods. AI leverages historical data to predict future trends, behaviors, and outcomes. Predictive models enable organizations to make proactive decisions, such as optimizing resource allocation or customizing product offerings.
📊 Time Series Analysis: AI algorithms can also perform time series analysis, which involves analyzing data points collected over a period to identify patterns and trends. This ability is particularly useful for forecasting future demand or identifying seasonality in sales data.
🗣️ Natural Language Processing (NLP): NLP enables AI systems to understand and analyze human language, allowing them to process and extract valuable insights from unstructured data sources such as social media posts, customer reviews, and news articles. This capability has greatly expanded the scope of data analytics, as previously inaccessible data sources can now be leveraged for business insights.
⏱️ Real-Time Analysis: With the ability to process large amounts of data quickly, AI allows for real-time analysis and decision-making, eliminating delays in obtaining insights. This is particularly useful in industries such as finance, where timely decisions can make a significant impact.
The Synergy Between AI and Data Analytics
The intersection of data analytics and artificial intelligence is a fascinating area that drives innovation and transformation across various industries.
AI complements data analytics by introducing a layer of intelligence that enables more nuanced and refined interpretation. As we learned in the section above, AI's intelligence, predictive capabilities and automation enhances analytical processes, enabling organizations to extract deeper insights from their data and move with agility. However, this is not a one-sided relationship. AI systems depend on data analytics to enhance and refine their capabilities. Without data analytics, AI would lack a blueprint to follow and improve upon. Using vast amounts of data and data analysis methods, AI technologies can be meticulously programmed to deliver precise forecasts and informed decisions. Moreover, data analytics plays a crucial role in enhancing AI algorithms by detecting and correcting biases and errors. Through thorough data examination, data scientists can pinpoint and amend biases within the training data, ensuring AI systems perform optimally. Furthermore, data analysis can reveal errors or inconsistencies, significantly improving the accuracy and dependability of AI models.
At the end of the day, without data analytics, there would be no AI data analysis tools. And without AI data analysis tools, data analytics would never have been able to make the monumental leap it has today.
AI’s Role in Future Data Analytics Trends
AI has already transformed data analytics, and it will continue to do so in the years to come. As technology advances, AI data analysis will become more sophisticated, enabling even more precise insights and predictions. Here are some future trends we can expect to see in the intersection of AI and data analytics:
Increased Use of Natural Language Processing: With the increasing amount of unstructured data available, the role of NLP in data analytics will only grow. AI-driven systems that can analyze and extract insights from text, audio, and video sources will become more advanced, enabling organizations to gain deeper understanding and valuable insights from a wide range of data.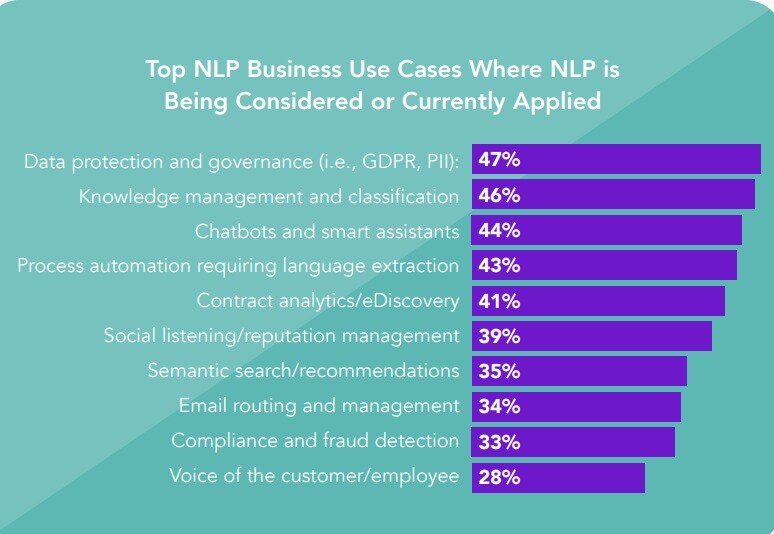
A 2023 survey conducted by expert.ai revealed that 77% of companies were expected to increase their investment in Natural Language Processing (NLP) projects over the following 12-18 months.
- Expanded Use of Advanced Analytics: As AI continues to evolve, so will its ability to handle complex analytical processes such as predictive modeling, time series analysis, and clustering. This will enable organizations to delve even deeper into their data and uncover new patterns and insights.
- Greater Adoption of AI in Decision-Making Processes: As AI becomes more accurate and reliable, it will play an increasingly important role in decision-making processes. Organizations will rely on AI-driven insights to make strategic decisions, leading to more efficient and effective outcomes.
- Further Exploration of Generative AI: Generative AI is already taking over. According to a McKinsey Global Survey, within a year of introducing generative AI tools, one-third of the surveyed organizations started using generative AI regularly in at least one part of their business.
Source: McKinsey
Its ability to generate predictive models, simulate scenarios, and discover unique solutions will continue to significantly impact AI data analysis. Gartner forecasts a significant growth in the application of generative AI for producing synthetic data. This advancement eases the challenge of acquiring real-world data, enabling the efficient training of machine learning models. Gartner estimates that 60% of data used for AI will be synthetic, crafted to mimic reality, envision future scenarios, and mitigate the risks associated with AI.
- Integration with IoT Devices: The Internet of Things (IoT) is a rapidly growing field that generates vast amounts of data. AI will play a crucial role in processing and analyzing this data, enabling organizations to make sense of the massive influx of information from various IoT devices. This will open up new opportunities for data-driven decision-making and insights.
- Larger Focus on Ethical Considerations: As AI continues to advance, ethical considerations surrounding its use in data analytics will become more critical. Organizations will need to ensure that their AI systems are unbiased and transparent, addressing concerns about privacy and potential discrimination. This may lead to the development of ethical standards and regulations for AI in data analytics.
As we can see, the future of data analytics is closely intertwined with AI. With its ability to process and analyze vast amounts of data quickly and accurately, AI will continue to enhance and advance data analytics, opening up new possibilities for businesses across all industries.
Top 7 AI Tools for Data Analysis
Looking for the best AI tool for data analytics? We've got you covered. Here are some of the top AI-powered tools used for data analysis today:
- Microsoft Power BI
- Tableau
- RapidMiner
- Qlik
- KNIME
- Polymer
- Julius AI
Comprehensive Review of the Best AI Tools for Data Analytics
Microsoft Power BI
One of the top tools for AI data analysis, Microsoft Power BI is a data simplification powerhouse. It has the capability to link with various data sources, enable quick analysis, and produce captivating, visually appealing reports that convert into significant business insights. This cloud-based tool provides organizations with an intuitive and user-friendly interface for data visualization, data preparation, reporting, and predictive modeling. Power BI, accessible as a SaaS platform, desktop application, and mobile app, offers a comprehensive view of business data to facilitate seamless team collaboration. Positioned within Microsoft's extensive suite of business applications, it frequently operates alongside Excel, Teams, and SharePoint. This not only allows for a seamless flow of data to take place, but also enables the creation of unified reports and dashboards and fosters a cohesive collaboration environment. Since Power BI is part of the Microsoft ecosystem, it adheres to the same security measures. Your data remains protected through features like Azure Active Directory integration and Row-Level Security (RLS). RLS ensures that users see only the data relevant to their roles, maintaining confidentiality and compliance.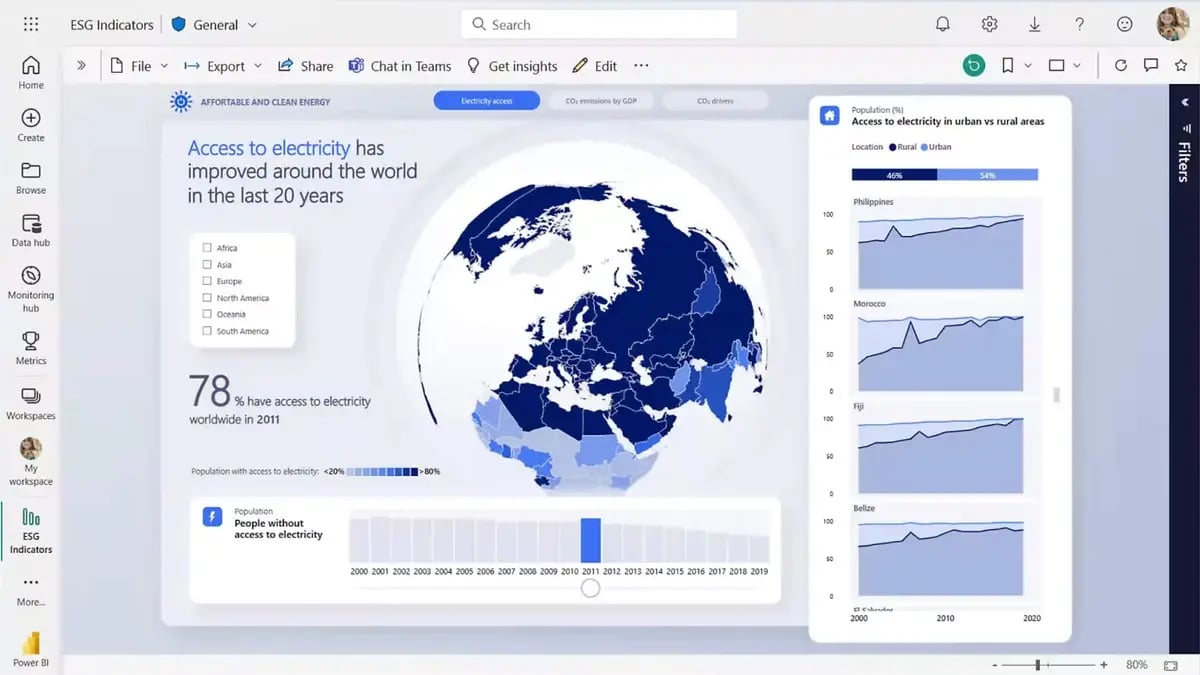
- Enables the creation of comprehensive, customizable and interactive reports and dashboards.
- Incorporates support for machine learning models and AI-driven capabilities to enhance data analysis.
- Facilitates data importation from a wide range of sources.
- Connects to various data sources and provides real-time updates through live data streams and trackable KPIs.
- Integrates seamlessly with Azure to transform data into a strategic asset.
At ProServeIT, we help organizations build a data-driven culture with Microsoft Power BI. Whether it's implementing this AI tool or elevating its usage, our experts are here to shape your data analytics journey.
Learn more about Power BI through our blog.
Tableau
Tableau stands out as a premier data visualization software, offering users the ability to craft interactive dashboards, charts, and maps with an easy-to-use drag-and-drop interface. This facilitates the simplification of exploring complex data sets. Its compatibility with numerous data sources, including Excel, databases, and cloud applications, is enhanced by advanced features like predictive analytics and natural language processing. The integration with Salesforce CRM is a notable advantage, providing a smooth transition between customer data and actionable insights, which proves beneficial for teams in sales, marketing, and customer service. Moreover, Tableau's AI and machine learning capabilities significantly enhance the analytics experience, positioning it as an essential tool for both analysts and novices, streamlining data exploration and the decision-making process. 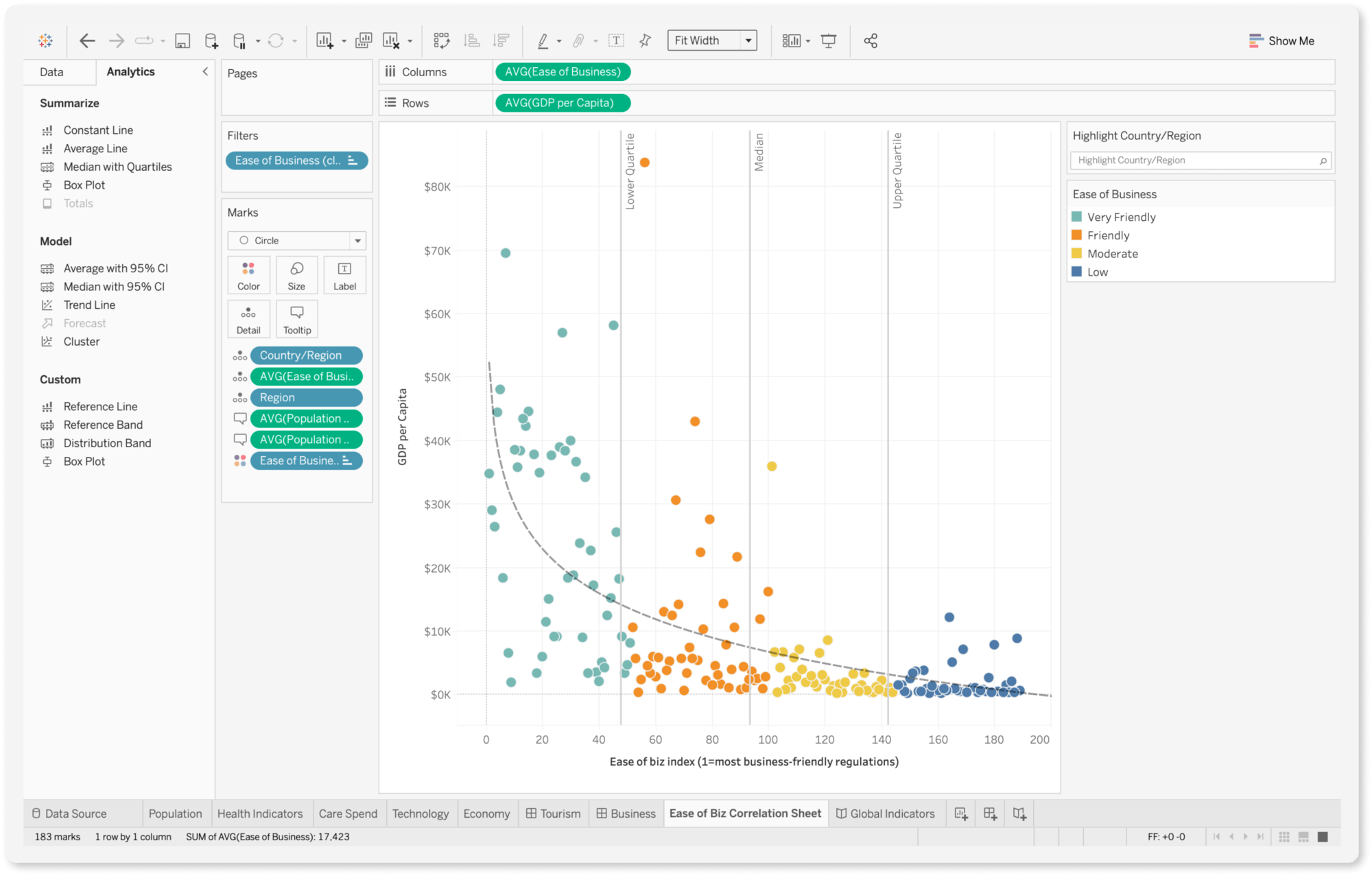
Source: Tableau
What makes Tableau a standout?
- Offers robust and customizable visualization options, including bar charts, heat maps, and tree maps.
- Facilitates storytelling through interactive dashboards.
- Provides real-time data updates for effective decision-making.
- Has a strong community support system with a variety of resources such as webinars, forums, and user groups.
RapidMiner
RapidMiner is recognized as a formidable data science platform that skillfully merges machine learning, predictive analytics, and AI to deliver valuable insights via a user-friendly interface, accessible even to non-technical users. It stands out with its impressive repertoire of over 1500 built-in machine learning algorithms, positioning it as a comprehensive solution for data mining, model development, and a spectrum of data analysis tasks including preparation and visualization. Its versatility is evident in its application across a variety of industries such as manufacturing, life sciences, energy, and healthcare, proving particularly advantageous for professionals dealing with previously mined or historical data. While it may exhibit certain constraints in feature engineering and selection, RapidMiner compensates with its powerful graphical programming language and an extensive marketplace for add-ons, establishing it as a potent AI data analysis tool for researchers and data scientists from diverse fields.
Source: RapidMiner
- AI-driven automation capabilities that enhance the speed and accuracy of data analysis.
- Powerful visualizations for effective communication of insights.
- Integrates with other popular tools such as Tableau and Power BI.
Qlik
Qlik is recognized as a robust business intelligence and data analytics platform that stands out for its use of AI and machine learning to facilitate real-time insights. The platform is user-friendly, offering straightforward functionality for the creation of data visualizations and dashboards. What sets Qlik apart is its unique associative engine, which seamlessly connects data elements from various sources, making it easy for users to explore and discover hidden patterns. Qlik Sense, a key component of the Qlik ecosystem, elevates data analysis by providing AI-generated insights, automated data preparation, and natural language interaction. Its capabilities extend to predictive modeling and machine learning, thereby bolstering effective decision-making. The platform's high-performance SaaS and hybrid cloud architecture offer organizations of any size remarkable analytics performance and flexibility. This enables comprehensive predictive analytics capabilities, available in the cloud, on-premises, or through SaaS, thereby affirming Qlik as a powerful tool for actionable insights.
Source: Qlik
What makes Qlik a standout?
- Robust associative data model that connects all data elements.
- AI-driven capabilities such as predictive analytics and natural language processing.
- User-friendly interface with drag-and-drop functionality for visualizations.
KNIME
KNIME is an exceptional open-source data analytics platform that skillfully merges visual programming, data mining, and machine learning, enabling users to create custom workflows for comprehensive data analysis. It boasts an extensive array of features such as data blending, transformation, aggregation, and visualization, making it a versatile tool for data analysts. The platform's seamless integration with multiple data sources, coupled with its capability to process both structured and unstructured data, positions it as an invaluable asset for managing and analyzing large datasets. Its collaborative framework enhances teamwork, by allowing multiple users to work on the same projects, thereby enhancing knowledge sharing and decision-making across various departments. With over 2000 modules and a continuously expanding community of contributors, KNIME is advancing as a powerful, flexible AI data analysis tool that caters to the needs of data-driven organizations.
Source: KNIME
- Open-source platform with a growing community of contributors.
- Powerful data processing capabilities, including handling both structured and unstructured data.
- Flexible and customizable workflows for various data analysis needs.
Polymer
Polymer is touted as a revolutionary AI-driven data analytics platform that merges machine learning, deep learning, and natural language processing techniques to deliver a comprehensive data understanding. It stands out for its advanced predictive modeling capabilities, allowing for the building of accurate and scalable models suitable for a variety of use cases. One of the platform's key strengths is its powerful feature engineering and selection tools, which greatly simplify the creation of effective features from complex datasets, thus easing the process of model training and deployment. Furthermore, its proficiency in natural language processing enables the extraction of valuable insights from unstructured data sources, such as text and images, offering users a holistic view of their data. Polymer focuses on being easy to understand and explain, making it perfect for companies that want to make smart decisions based on data and follow ethical AI rules.
Source: Polymer
- Advanced AI capabilities such as natural language processing and deep learning.
- Emphasis on interpretability and explainability for ethical AI practices.
- Comprehensive data understanding through feature engineering and selection.
Julius AI
An advanced AI data analysis tool, Julius AI is a cloud-based platform that can read and comprehend text data in multiple languages, making it ideal for analyzing large datasets quickly. With its natural language processing capabilities, Julius AI can extract insights from unstructured data such as news articles, social media posts, and customer feedback. The platform also offers customizable dashboards, real-time sentiment analysis, and can integrate with various data sources like Google Analytics, Salesforce, and Zendesk.
Source: JuliusAI
What makes Julius AI a standout?- Has the ability to analyze large amounts of unstructured data at an efficient pace.
- Possesses multilingual capabilities for analyzing text data in multiple languages.
- Provides customizable dashboards and real-time sentiment analysis.
- Supports various data file formats, including spreadsheets, Google Sheets, and Postgres databases.
Conclusion
In conclusion, the symbiotic relationship between AI and data analytics enhances data analysis precision and efficiency, playing a crucial role in the digital age. AI data analysis is vital for businesses, enabling informed decisions through accurate predictions and deep insights, significantly boosting their agility and competitiveness. Integrating AI data analysis tools into business intelligence workflows has revolutionized data handling, enabling faster decision-making and a deeper understanding of complex patterns in large datasets.
The synergy between AI and data analytics is driving innovation, with trends showing a growing reliance on these technologies to improve analytical capabilities. The rise of Natural Language Processing (NLP), AI's integration in decision-making, and its connection with IoT devices are rapidly evolving the data analytics landscape. Ethical considerations and developing standards will ensure this progress benefits everyone.
In this transformative period, it is imperative for companies to commence their AI journeys and foster a robust data-driven culture to enhance their bottom line. Leveraging the expertise of partners, like ProServeIT, presents a formidable solution for organizations aiming to stay at the forefront of this wave.
Ultimately, engaging with this AI wave is not merely an option but a necessity for organizations looking to evolve and thrive in the modern, data-centric world.
Make the Most of Your Data with ProServeIT
Whether you're ready to begin your data and analytics journey or even looking to level up your current data analysis processes, ProServeIT is at your service. As a Microsoft Solutions Partner in Data & AI, our experts are here to support your organization's unique data needs. Our team of certified Data & Analytics professionals will collaborate with you to develop a roadmap for success that matches your business goals with your IT strategy. We'll assist in speeding up your digital transformation, making sure your data and analytics are dependable, secure, budget-friendly, and scalable. Start your data and analytics journey with us today.
If you want to move right ahead with integrating our Microsoft AI solutions, like Microsoft Power BI, into your organization's infrastructure, look no further. We'll help you unlock the benefits of Power BI and turn your data into valuable insights so that you can foster a data-driven culture with ease. Reach out to us today and learn how ProServeIT can help you make the most of your data.